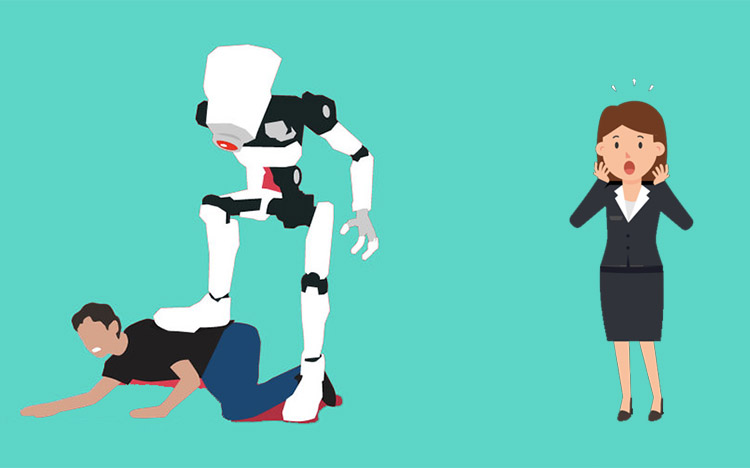
10 Common Misconceptions about Artificial Intelligence (AI)
Our lives are now affected by AI. Still, there are a lot of misconceptions about Artificial Intelligence. Maybe because of politics, or because of Science Fiction. Here are some of them.
Misconception 1: Very smart AI already exists
One of the most common misunderstandings is that ‘very clever AI already exists’, or that ‘human-like intelligence’ is achieved. While the long term goal is to achieve a general purpose artificial intelligence suitable for all kinds of jobs, it is a far off goal. AI is a buzzword of the present, but a general purpose AI as imagined in Sci-Fi movies belongs only to a distant future. It is also important for companies to separate Sci-Fi from reality and not try to fool their consumers and investors about their AI capabilities.
Misconception 2: Great AI can be developed quickly
In February 2011, Watson won the challenge in the quiz show“ Jeopardy! ”. Also, in March 2016, AlphaGo defeated Lee Sedol in the game of Go. In light of these, there is a popular notion that AI can great things immediately. However, it is necessary to understand that it is not so simple.
These are applications of machine learning and deep learning. Even so, the introduction of these technologies does not mean that other similar things can be realized immediately.
Along with great technologies, there must be great engineers to work on them. Companies and individuals both need to first understand that it is wrong to think that “anyone can do something quickly if AI is introduced”. As of now, AI technologies are similar to babies or children if you compare them with humans. So it is important to remember that the developing “skills” of the ‘child’ is also important for successful development.
It might take at least 10 years or more to reach a level at which artificial intelligence can do anything. 80% of the existing work and technologies will be challenged by AI technology. It won’t happen overnight, but it will certainly happen. Competition in job industries will decline due to a lack of competent human resources.
Misconception 3: There is a single technology called AI
There is a lot of research on artificial intelligence. Also, there is a wide range of technical fields related to AI. So, there is no single thing called “AI”. Deep learning, which is now attracting attention, is yet another technology, which is a part of machine learning. Machine Learning itself is a technology for artificial intelligence.
Misconception 4: AI is a software technology
This may depend on how you use the term “software technology”. You will often see “R, Python, Hadoop, Spark” listed as examples of the technologies required to run AI. This is correct in one way, but not entirely.
These are just tools. So by using them, we can meet the requirements but it is not sufficient. In AI technology, these are only some elements which help in problem-solving, data preparation, method selection, implementation, etc. We can implement AI with “R, Python, Hadoop, Spark”, and so on. However, you must realize that these are not the only things we need for AI, nor are these the only way to implement AI. So, the perception that “AI is a software technology” is wrong (or at least misleading).
Misconception 5: Efficiency increases soon after introducing AI.
It depends on how well you can set the problem. It is easy to understand the result if the winners and losers are obvious in a game like Go. On the other hand, if you look at the field of business, it is not surprising that the results are hard to understand.
For example, you can assume that that sales forecasting has made it possible to set a problem and have high precision (though it is quite difficult itself). However, even if you can forecast the sales, you should not necessarily be happy.
Consider these questions. What we want to do is to improve sales. What is the basis of the prediction? Whether it is possible to manipulate the variables? Whether it is expensive for the operation? Supposing it goes well, is it satisfactory? Is it effective to meet the costs?
These are some difficult questions to answer even if you have the help of AI.
Misconception 6: Deep Learning is very powerful
It depends on the problem. It is related to the previous misunderstanding. For example, let’s say that you can forecast sales with deep learning.
So what do you focus on if you want to improve sales? Deep Learning does not have an answer for that.
For example, if you forecast sales using multiple regression analysis and then sales = 1.5 x number of business visits + … + and so on, you can see that if you increase the number of business visits, sales will rise.
With this problem setting, multiple regression analysis might be better than deep learning.
Misconception 7: “Unsupervised learning” is better than “Supervised learning” because you do not have to teach it.
Unsupervised learning and supervised learning are fundamentally different.
Basically, you use unsupervised learning to do clustering (to find similar things) or dimensionality reduction (reducing a large number of variables to only important factors).
Supervised learning is useful for Regression (prediction) and classification (discrimination). However, you cannot do these things with unsupervised learning.
Hence, both methods have their own usages which are fundamentally different. So it is wrong to compare them with each other like this.
Misconception 8: You can choose algorithms like you can choose computer languages
As for “algorithm”, or the analysis method, it depends on the type of data you have and on the answers you want. It also depends on the problem setting. So, depending on your objectives and situation, you may or may not have a choice.
You can refer to 10 Important Algorithms in Machine Learning for a better idea at the type of popular algorithms available.
Misconception 9: There are AI applications that anyone can use immediately.
There are many tutorials for learning about AI. However, there are not enough ready-to-use AI applications that can solve problems.
Also, it is true that the development environments are maintained as libraries or as API. So you can use them writing all the programs from scratch as before. Still, so the threshold is lowered. However, there are very few applications which can be deployed immediately or are meant for the layman.
Misconception 10: After all, AI is useless since we don’t have much use for it yet
AI is still developing.
Although it is great at playing Go and Chess, speech recognition has improved and so on. We must keep in mind that even elementary school children can not say exactly say what they want to say.
The areas where computers are good and the areas where people are unique are very different.
Go is actually “easy” for a computer. Even if you do not know what the other party thinks, all the results are on the board and the rules are obvious. All this information is revealed and the rules are obvious when the computer is good. However, the reason why it was a breakthrough is that the amount of calculation is huge.
If you think of it, the computational complexity is at most a problem setting. Naturally, AI is very good at it. It would be impossible for humans to classify 1,000,000 photos, it might even be a form of torture. However, computers can solve such problems easily with deep learning.
AI, though promising, is at a larval stage as of now. Still, we must realize that the metamorphosis itself might happen in a matter of a few years. The development of technology is exponential in growth.